Combined VMD-Morlet Wavelet Filter Based Signal De-noising Approach and Its Applications in Bearing Fault Diagnosis
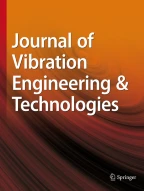
Rolling element bearings are an essential part of rotating machinery. Sudden bearing failure may lead to catastrophic machine failure. Early bearing fault detection is essential to avoid machine failure. Vibration data received from bearings typically contain impulsive fault information. The characteristics acquired from the vibration signals generated by bearings are primarily used to identify bearing defects. The derived features might not be able to accurately pinpoint the failure’s timing due to background noise in the observed vibration signal. External noise reduction from the vibration signal is essential for extracting important features for effective fault diagnosis. A helpful de-noising method at present is variational mode decomposition (VMD). However, the VMD method alone may not eliminate the noise from the vibration data.
Methods
The present work proposes a methodology for noise reduction combining VMD and an optimized Morlet filter. Initially, the signal is split using the VMD approach into various intrinsic mode functions (IMF), and the most efficient IMF is chosen using the maximum kurtosis criterion. Next, the golden ratio optimization method (GROM) based Morlet wavelet filter is applied to the effective IMF for reducing unwanted noise. The convolutional neural network (CNN) technique is then employed to identify the bearing defects.
Conclusion
The proposed approach is tested upon bearing simulation datasets, bearing experimental datasets, gearbox experimental datasets, and sound datasets to validate its efficiency. The validation of the proposed algorithm using gear and sound datasets indicates its broad applicability.
This is a preview of subscription content, log in via an institution to check access.
Access this article
Subscribe and save
Springer+ Basic
€32.70 /Month
- Get 10 units per month
- Download Article/Chapter or eBook
- 1 Unit = 1 Article or 1 Chapter
- Cancel anytime
Buy Now
Price includes VAT (France)
Instant access to the full article PDF.
Rent this article via DeepDyve
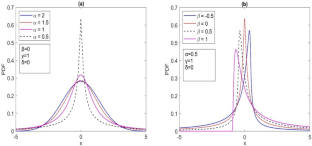
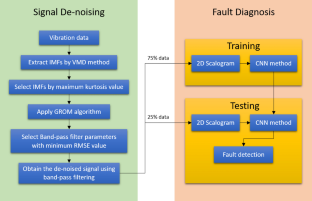
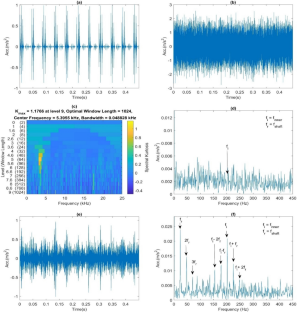
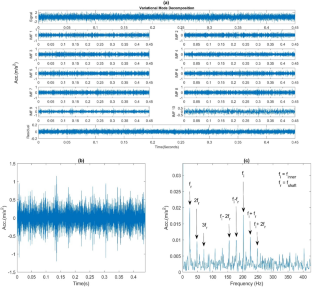
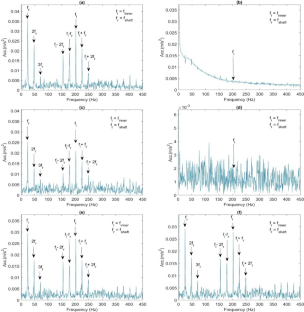
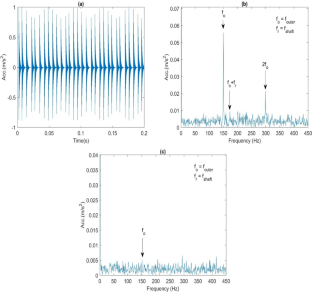
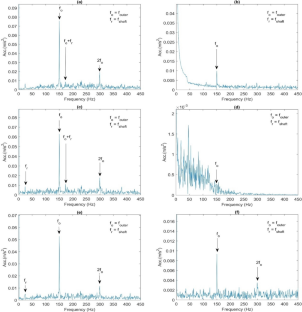
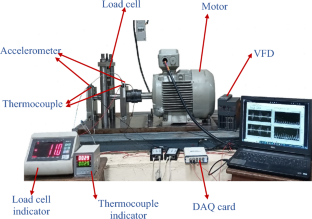
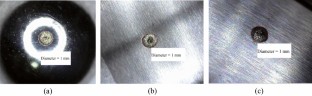
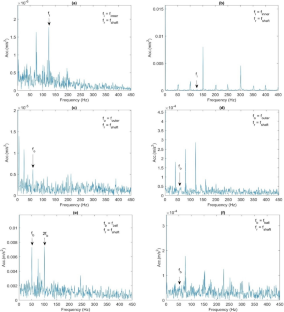
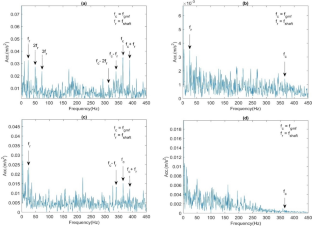
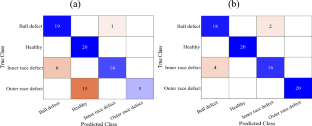
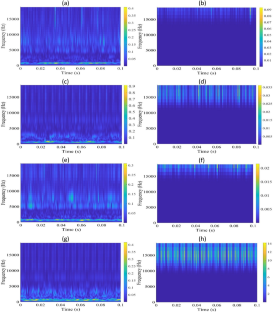
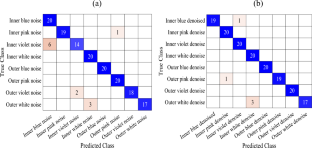
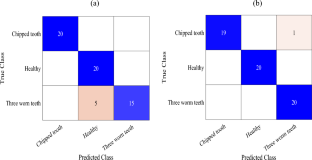
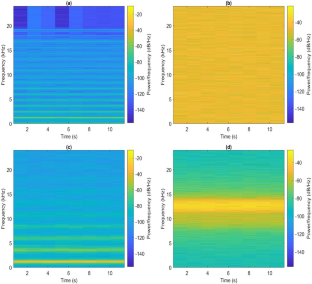
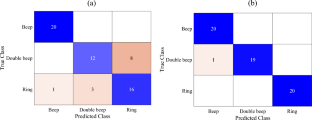
Similar content being viewed by others
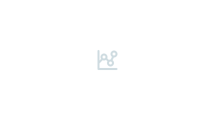
An improved bearing fault diagnosis method based on variational mode decomposition and adaptive iterative filtering (VMD-AIF)
Article 01 April 2023
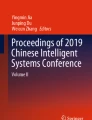
A Novel Bearing Intelligent Fault Diagnosis Method Based on Modified VMD and 1-D CNN
Chapter © 2020
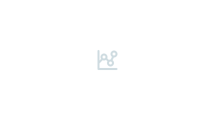
CEEMDAN and OWMRA as a hybrid method for rolling bearing fault diagnosis under variable speed
Article 11 September 2017
Abbreviations
Ball pass frequency inner
Ball pass frequency outer
Ball spin frequency
Empirical mode decomposition
Ensemble empirical mode decomposition
Empirical WAVELET TRANSFORM
Fast Fourier transform
Gear mesh frequency
Golden ratio optimization method
High-frequency resonance technique
Intrinsic mode function
Kernel density estimation
Probability density function
Root mean square error
Simulated inner signal
Simulated outer signal
Support vector machine
Variational mode decomposition
References
- Buchaiah S, Shakya P (2022) Bearing fault diagnosis and prognosis using data fusion based feature extraction and feature selection. Meas J Int Meas Confed 188(2021):110506 ArticleGoogle Scholar
- Liu X, Xia L, Shi J, Zhang L, Wang S (2023) Fault diagnosis of rolling bearings based on the improved symmetrized dot pattern enhanced convolutional neural networks. J Vib Eng Technol 12:1–12 Google Scholar
- Zheng X, Liu X, Zhu C, Wang J, Zhang J (2023) Fault diagnosis of variable speed bearing based on EMDOS-DCCNN model. J Vib Eng Technol. https://doi.org/10.1007/s42417-023-01085-2ArticleGoogle Scholar
- Ruan D, Zhang F, Zhang L, Yan J (2023) Optimal modifications in CNN for bearing fault classification and adaptation across different working conditions. J Vib Eng Technol. https://doi.org/10.1007/s42417-023-01106-0ArticleGoogle Scholar
- Sun Y, Li S, Wang X (2021) Bearing fault diagnosis based on EMD and improved Chebyshev distance in SDP image. Meas J Int Meas Confed 176:109100 ArticleGoogle Scholar
- Abdelkader R, Kaddour A, Derouiche Z (2018) Enhancement of rolling bearing fault diagnosis based on improvement of empirical mode decomposition denoising method. Int J Adv Manuf Technol 97(5–8):3099–3117 ArticleGoogle Scholar
- Sahu PK, Rai RN (2022) Fault diagnosis of rolling bearing based on an improved denoising technique using complete ensemble empirical mode decomposition and adaptive thresholding method. J Vib Eng Technol. https://doi.org/10.1007/s42417-022-00591-zArticleGoogle Scholar
- Buchaiah S, Shakya P (2022) Automatic incipient fault detection and health state assessment of rolling element bearings using pruned exact linear time method. JVC/J Vib Control 29:5148–5160 ArticleGoogle Scholar
- Mishra C, Samantaray AK, Chakraborty G (2017) Rolling element bearing fault diagnosis under slow speed operation using wavelet de-noising. Meas J Int Meas Confed 103:77–86 ArticleGoogle Scholar
- Wang Z, Zhang Q, Xiong J, Xiao M, Sun G, He J (2017) Fault diagnosis of a rolling bearing using wavelet packet denoising and random forests. IEEE Sens J 17(17):5581–5588 ArticleGoogle Scholar
- Dwyer F (1983) Detection of non-Gaussian signals by frequency domain kurtosis estimation. In: ICASSP’83. IEEE Int. Conf. Acoust. Speech, Signal Process., vol. 8, pp. 607–610
- Antoni J (2006) The spectral kurtosis: a useful tool for characterising non-stationary signals. Mech Syst Signal Process 20(2):282–307 ArticleGoogle Scholar
- Antoni J (2007) Fast computation of the kurtogram for the detection of transient faults. Mech Syst Signal Process 21(1):108–124 ArticleGoogle Scholar
- Antoni J, Randall RB (2006) The spectral kurtosis: application to the vibratory surveillance and diagnostics of rotating machines. Mech Syst Signal Process 20(2):308–331 ArticleGoogle Scholar
- Wang D, Tse PW, Tsui KL (2013) An enhanced Kurtogram method for fault diagnosis of rolling element bearings. Mech Syst Signal Process 35(1–2):176–199 ArticleGoogle Scholar
- Lei Y, Lin J, He Z, Zi Y (2011) Application of an improved kurtogram method for fault diagnosis of rolling element bearings. Mech Syst Signal Process 25(5):1738–1749 ArticleGoogle Scholar
- Xu Y, Zhang K, Ma C, Cui L, Tian W (2019) Adaptive kurtogram and its applications in rolling bearing fault diagnosis. Mech Syst Signal Process 130:87–107 ArticleGoogle Scholar
- Mishra SK, Shakya P, Babureddy V, Ajay-Vignesh S (2021) An approach to improve high-frequency resonance technique for bearing fault diagnosis. Meas J Int Meas Confed 178:109318 ArticleGoogle Scholar
- Afia A, Rahmoune C, Benazzouz D (2018) Gear fault diagnosis using Autogram analysis. Adv Mech Eng 10(12):1–11 ArticleGoogle Scholar
- Dragomiretskiy K, Zosso D (2014) Variational mode decomposition. IEEE Trans Signal Process 62(3):531–544 ArticleMathSciNetGoogle Scholar
- Nematollahi AF, Rahiminejad A, Vahidi B (2020) A novel meta-heuristic optimization method based on golden ratio in nature, vol 24. Springer, Berlin Heidelberg Google Scholar
- Yu G, Li C, Zhang J (2013) A new statistical modeling and detection method for rolling element bearing faults based on alpha-stable distribution. Mech Syst Signal Process 41(1–2):155–175 ArticleGoogle Scholar
- Shao M, Nikias CL (1993) Signal processing with fractional lower order moments: stable processes and their applications. Proc IEEE 81(7):986–1010 ArticleGoogle Scholar
- Middleton D (1999) Non-Gaussian noise models in signal processing for telecommunications: new methods and results for Class A and Class B noise models. IEEE Trans Inf Theory 45(4):1129–1149 ArticleMathSciNetGoogle Scholar
- Bouvet M, Schwartz SC (1989) Comparison of adaptive and robust receivers for signal detection in ambient underwater noise. IEEE Trans Acoust 37(5):621–626 ArticleGoogle Scholar
- Xiaohu G, Guangxi Z, Yaoting Z (2004) On the testing for alpha-stable distributions of network traffic. Comput Commun 27(5):447–457 ArticleGoogle Scholar
- Liu J, Wang W, Golnaraghi F (2008) An extended wavelet spectrum for bearing fault diagnostics. IEEE Trans Instrum Meas 57(12):2801–2812 ArticleGoogle Scholar
- Yang WX, Ren XM (2004) Detecting impulses in mechanical signals by wavelets. EURASIP J Appl Signal Processing 2004(8):1156–1162 Google Scholar
- Rafiee J, Rafiee MA, Tse PW (2010) Application of mother wavelet functions for automatic gear and bearing fault diagnosis. Expert Syst Appl 37(6):4568–4579 ArticleGoogle Scholar
- He W, Jiang ZN, Feng K (2009) Bearing fault detection based on optimal wavelet filter and sparse code shrinkage. Meas J Int Meas Confed 42(7):1092–1102 ArticleGoogle Scholar
- Key S (2000) Feature extraction based on Morlet wavelet and its application for mechanical fault. J Sound Vib 234:135–148 ArticleGoogle Scholar
- Nikolaou NG, Antoniadis IA (2002) Demodulation of vibration signals generated by defects in rolling element bearings using complex shifted Morlet wavelets. Mech Syst Signal Process 16:677–694 ArticleGoogle Scholar
- Saravanan N, Siddabattuni VNSK, Ramachandran KI (2008) A comparative study on classification of features by SVM and PSVM extracted using Morlet wavelet for fault diagnosis of spur bevel gear box. Expert Syst Appl 35:1351–1366 ArticleGoogle Scholar
- Li H, Liu T, Wu X, Chen Q (2020) An optimized VMD method and its applications in bearing fault diagnosis. Meas J Int Meas Confed 166:108185 ArticleGoogle Scholar
- Shakya P, Darpe AK, Kulkarni MS (2017) Bearing damage classification using instantaneous energy density. JVC/J Vib Control 23(16):2578–2618 ArticleGoogle Scholar
- McFadden P, Smith J (1984) Model for the vibration produced by a single point defect in a rolling element bearing. J Sound Vib 96:69–82 ArticleGoogle Scholar
- Zamanian AH (2014) Experimental dataset for gear fault diagnosis. Exp. data, pp 2–3
- LeCun Y, Bengio Y (1995) Convolutional networks for images, speech, and time series. Handb Brain Theory Neural Netw 3361:255–258 Google Scholar
- Amin A, Bibo A, Panyam M, Tallapragada P (2023) Wind turbine gearbox fault diagnosis using cyclostationary analysis and interpretable CNN. J Vib Eng Technol 12:1–11 Google Scholar
- Ribeiro-Junior RF, dos Santos-Areias IA, Campos MM, Teixeira CE, da Silva LEB, Gomes GF (2022) Fault detection and diagnosis in electric motors using convolution neural network and short-time Fourier transform. J Vib Eng Technol 10(7):2531–2542 ArticleGoogle Scholar
- Yuan Z, Ma Z, Li X, Cui Y (2023) Speed adaptive graph convolutional network for wheelset-bearing system fault diagnosis under time-varying rotation speed conditions. J Vib Eng Technol 12:1–12 Google Scholar
- Liu X, Zhang Z, Meng F, Zhang Y (2022) Fault diagnosis of wind turbine bearings based on CNN and SSA–ELM. J Vib Eng Technol 11:1–17 Google Scholar
Author information
Authors and Affiliations
- Engineering Asset Management Group, Department of Mechanical Engineering, Indian Institute of Technology Madras, Chennai, 600036, India Akshay Rajendra Patil, Sandaram Buchaiah & Piyush Shakya
- Akshay Rajendra Patil